How machine learning is revolutionizing demand planning - and what it means for you?
In this blog post our Lead Data Scientist Christian explains how machine learning is transforming demand forecasting in various industries. He explores the shift from traditional models to machine learning methods and the main model types relevant to demand forecasting. And highlights the advantages of using machine learning in demand forecasting.

by Christian Montes Schütte, PhD | Assoc Prof, April 28, 2023
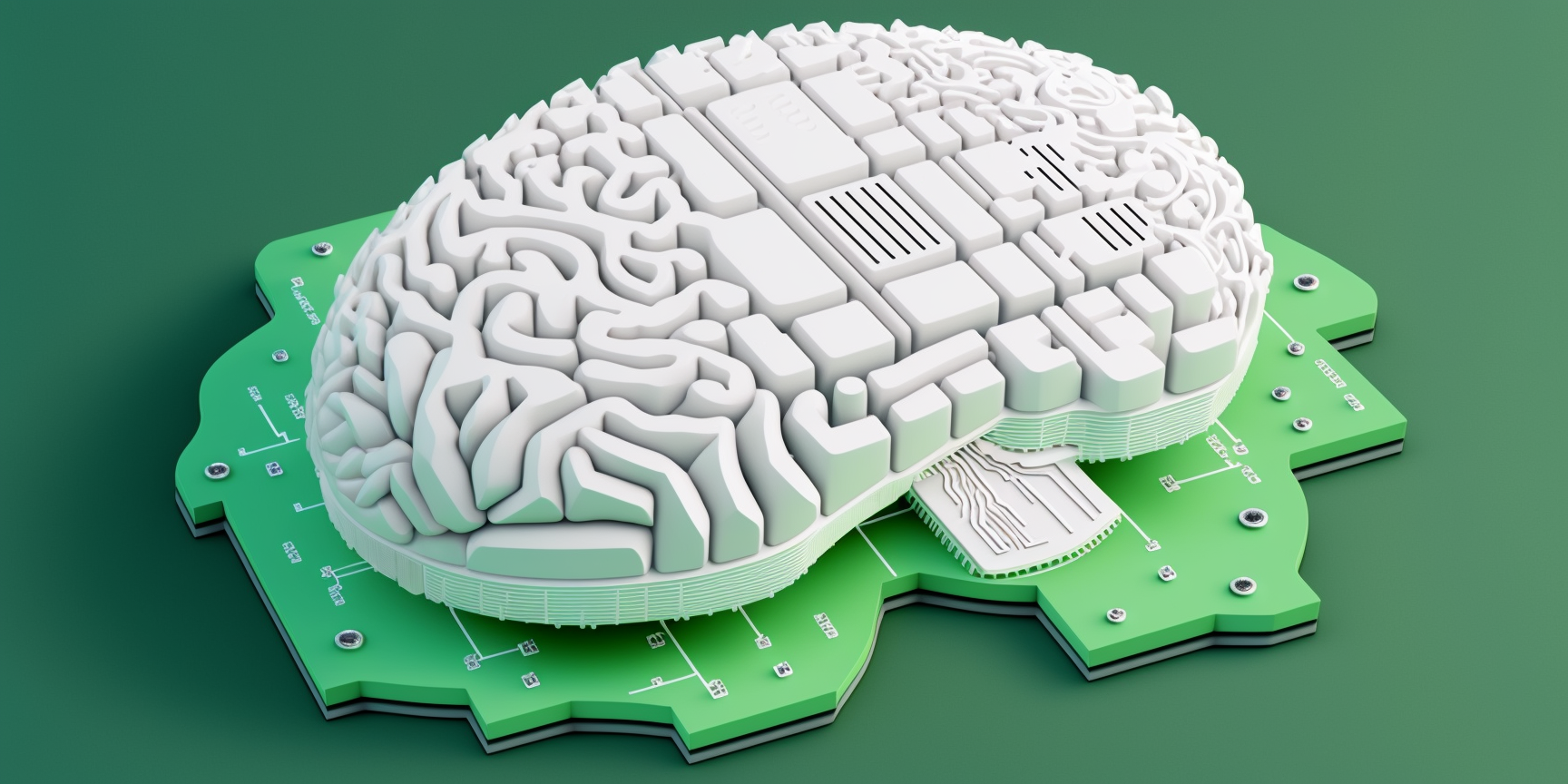
Introduction
In today's digital age, is revolutionizing various industries by providing innovative solutions to complex problems. plays a critical role in driving sales, managing inventory, and ensuring customer satisfaction across a wide range of sectors. Traditionally, many businesses have relied on univariate models to make predictions based on historical data. However, as the amount of metadata on customers and products has grown, there's a growing potential for industries to leverage machine learning methods to enhance their forecasting capabilities. In this blog post, we'll explore what machine learning is, the main model types relevant to demand forecasting, and how these techniques can improve predictive accuracy for businesses across diverse sectors.
Understanding Machine Learning
To understand the potential of machine learning for , it's essential to first grasp the concept of machine learning itself. In plain words, machine learning is a subset of artificial intelligence (AI) that allows computers to learn from data, recognize patterns, and make predictions or decisions without being explicitly programmed to do so. The more data a machine learning algorithm is exposed to, the better it becomes at identifying underlying patterns and relationships, ultimately leading to improved predictions over time. In the context of demand forecasting, machine learning can process vast amounts of information, including , customer demographics, and product details, to generate more accurate and timely forecasts that can significantly impact business performance.
The Shift from Traditional Methods to Machine Learning
As industries evolve and data becomes more abundant, the shift from traditional univariate time series models to machine learning methods for demand forecasting is gaining momentum. Univariate time series models rely solely on historical data to predict future trends, while machine learning techniques can leverage a wide range of variables, including customer and product metadata, to make more informed predictions. This transition enables businesses to gain a deeper understanding of the factors affecting demand, ultimately leading to more accurate and timely forecasts. For instance, by incorporating customer demographics and behavior patterns, machine learning models can better account for the influence of factors like seasonal trends, holidays, or promotional events on demand. Additionally, product metadata, such as pricing, availability, or product attributes, can be integrated into the forecasting process to capture the impact of these features on consumer preferences and purchasing decisions. By combining these data sources, machine learning models are better equipped to adapt to changing market conditions and anticipate fluctuations in demand, providing businesses with a competitive advantage in managing their resources and meeting customer needs.
Main Model Types Relevant to Demand Forecasting
Machine learning can be understood through three main model types relevant to demand forecasting: supervised learning, unsupervised learning, and reinforcement learning. Supervised learning is like teaching a child with a labeled picture book, while unsupervised learning is like asking a child to sort unlabeled toys based on their similarities. Reinforcement learning, on the other hand, is akin to teaching a child to ride a bike through trial and error. Among these, supervised and unsupervised learning models are more commonly used in demand forecasting.
Supervised Learning Models
Supervised learning models are essential in demand forecasting, as they learn from examples that include both input information (e.g., past sales, promotions, customer demographics) and corresponding results (e.g., future demand). Regression algorithms, which predict continuous values like sales or revenue, are a popular choice within the supervised learning techniques for demand forecasting. Some well-known regression methods are linear regression, , and . Linear regression is a straightforward approach that finds a straight line that best fits the data, allowing us to forecast future demand based on past information and other relevant factors. Decision trees are more flexible and can capture more complex relationships in the data. They work by repeatedly dividing the data into smaller groups based on the most important input factors, creating a tree-like structure where each ending point represents a demand forecast. This method provides an easily understandable visual representation of the factors influencing demand. Neural networks are more advanced techniques inspired by the way the human brain works. They consist of interconnected processing units that work together, allowing the model to learn complicated relationships between inputs and outputs. Although neural networks can be more challenging to set up and understand, they offer the potential for highly accurate demand forecasts, especially when working with large, intricate datasets.
Unsupervised Learning Models
Unsupervised learning models can also contribute to demand forecasting by uncovering hidden patterns or structures within the data. Clustering and dimensionality reduction algorithms are two key techniques within unsupervised learning. Clustering methods, like the popular k-means clustering, group similar data points together based on their characteristics, which can help reveal trends or customer segments that can inform demand forecasting. Dimensionality reduction algorithms, such as principal component analysis (PCA), simplify complex datasets by reducing the number of variables while retaining the most important information. These reduced variables can then be used as inputs in supervised learning models, like regression frameworks, to predict demand more accurately. By combining the strengths of both supervised and unsupervised learning models, businesses can create more robust demand forecasting solutions that account for a wider range of factors influencing demand.
Reinforcement Learning Models
Although reinforcement learning may not be the primary focus for e-commerce applications in demand forecasting, it still has potential applications in the broader context. Reinforcement learning involves an agent learning to make decisions based on feedback received from the environment in the form of rewards or penalties. This approach can be particularly useful for optimizing inventory management and supply chain strategies, as it allows businesses to learn from their actions and adapt their policies to maximize profits or minimize costs. For example, a reinforcement learning model could be used to determine or in an inventory management system, taking into account factors such as lead times, holding costs, and stockout costs. By continually learning from and adapting to changing market conditions, reinforcement learning can help businesses develop more efficient and responsive supply chain strategies that can complement their demand forecasting efforts.
Advantages of Machine Learning in Demand Forecasting
Machine learning offers several advantages for demand forecasting, making it an attractive alternative to traditional forecasting methods. One of the primary benefits is increased , as machine learning models can leverage a wide range of variables, including customer and product metadata, to make more informed predictions. This enables businesses to gain a deeper understanding of the factors affecting demand, ultimately leading to more accurate and timely forecasts. Another advantage is adaptability to changing trends, as machine learning models can continuously learn from new data and update their predictions accordingly. This feature allows businesses to respond more effectively to fluctuations in the market, ensuring they are always prepared for shifts in demand. Furthermore, machine learning models excel at handling complex relationships in data, capturing both linear and non-linear patterns that may be difficult to identify using traditional methods. This ability to handle complexity can lead to more nuanced demand forecasts that account for a broader range of factors influencing consumer behavior, allowing businesses to better plan their resources and meet customer needs.
Conclusion
In conclusion, machine learning has the potential to transform demand forecasting across various industries, providing businesses with more accurate, timely, and adaptable predictions. By leveraging the power of supervised and unsupervised learning models, companies can better understand the complex factors influencing demand and make more informed decisions about resource allocation, inventory management, and supply chain strategies. Furthermore, the adaptability of machine learning models ensures that businesses can stay ahead of market fluctuations and evolving trends. More accurate demand forecasting can also lead to reduced waste, minimize the so-called , and decrease net working capital requirements. As the digital landscape continues to grow, it's crucial for organizations to explore the benefits of machine learning for demand forecasting and harness its potential to gain a competitive advantage, enhance customer satisfaction, drive long-term success, and promote sustainable business practices.
Stay ahead of the curve with our expert insights!
Subscribe to Hakio blog for curated insights on innovative demand planning and forecasting techniques. Sign up now and supercharge your strategy with our expert tips delivered straight to your inbox.